In a recent publication, Peter Bönisch and Roman Inderst tackle the delicate issue of the evaluation of seemingly contradictory econometric evidence. Introducing the concept of severity measures, they propose a method to avoid the common obstacles plaguing the interpretation of seemingly conflicting empirical evidence through the practical example of financial damage estimation in follow-on cases. The topic discussed by Bönisch and Inderst is particularly important considering the practical aspects of litigation cases, as judges typically do not have an in-depth knowledge of econometrics. This may result sometimes in the unwarranted situation that they blindly disregard contradictory evidence by party experts as assumably non-liquet,1See practical examples of national judgments on competition private enforcement cases in which the judges rejected party-expert econometric evidence on inconsistency grounds: Bundesgerichtshof, 9 October 2018, KRB 10/17; Tribunal Administratif de Paris, 9 Janvier 2009, Requête N°9800111. and instead opt for other solutions such as their own independent estimation of damages.2See CJEU, Request for a preliminary ruling from the Juzgado de lo Mercantil n.o 3 de Valencia (Spain) lodged on 19 May 2021 — Tráficos Manuel Ferrer, S.L. and Other v Daimler AG, C-312/21, where the judge asked whether the power of the National Court to estimate the amount of damages enables those damages to be quantified independently on the grounds of a situation of information asymmetry or insoluble difficulties regarding quantification. Opinion of Advocate General Kokott on this question is set to be delivered on 22 September 2022.
How can expert estimation results be contradictory?
According to the authors, divergent empirical findings in estimating financial damages can typically be explained by multiple reasons. In the context of private damage litigation for competition law infringement, the main source of uncertainty often comes from the asymmetric information availability between the parties, which leads to divergent statistical data sets. Indeed, in such cases, the parties simply do not have the same level of access to the different sets of data available, under which circumstances it is difficult to reach true equality of arms between the claimant and the defendant. Thus, a strong asymmetry of information remains in favour of the defendant. Furthermore, conflicting estimated results can be explained by the differences in the underlying econometric model of each party, which is commonly based on conflicting assumptions, such as, for example, the structure of the model and the set of variables used in the estimation.
These differences lead, expectedly, to contradictory results. Because of these discrepancies, the opposing statistical estimates put forward by the two parties can be interpreted to be incompatible while, in essence, both pieces of evidence describe the same empirical phenomenon and reach opposite conclusions because of the uncertainty risks described above. Hence, Bönisch and Inderst screen the compatibility level of seemingly contradictory empirical results through the concept of the statistical severity of the furnished evidence.
Estimating infringement effects in private enforcement: the common procedure
In their publication, Bönisch and Inderst take a numerical example of a follow-on private action for damages. In this context, both parties submit expert testimony based on econometric estimations. In their hypothetical example, both the claimants and the defendants perform market assessments to quantify the damage per item resulting from the infringement (hereinafter, “the infringement effect”), yet the experts reach opposite conclusions: statistically insignificant damages of 1€ for the defendant versus statistically significant damages of 8€ for the claimant.
The authors point out that practitioners must be careful with the interpretation of statistical significance. In econometrics, a result is statistically significant if, with a “high enough” probability, the measured effect is real. In other words, it is highly probable that the impact does not occur only by chance (aka Type I error). There is no rule of thumb as to what probability is “high enough”, but empirical best practices use a cut-off point of 90% or 95%. Note that the usual statistical significance test is assessing the reliability of the results in comparison to a zero-effect (“null-hypothesis”), so we can tell by looking at the probability values whether the result is different from zero. However, could there be any other cutoff points? The article argues that exactly the testing vis-à-vis other cutoff points is the key to smoothing the differences between diametrically opposing results as in the example above.
Measuring severity levels of possible infringement effects
The statistical notion of “severity” was first introduced by Mayo and Spanos in 2006. The general idea behind a severity assessment is to test the statistical evidence at hand against an alternative hypothesis beyond the “general” null-hypothesis. Let’s take the authors’ example in which the plaintiff’s statistical evidence proved an 8€ damage estimation being significantly different from zero. Using the similar standard statistical calculations as before, the goal is now to measure the level of certainty of this evidence for values of other possible infringement effects x different than zero, such as 2€ or 6€. So, the general question on null-hypothesis testing whether the measured impact is significantly different from zero is replaced with the question whether the measured impact is significantly different from 2€ or 6€. Naturally, the further away we define (positive) x from zero, the lower the probability that the estimated damage of 8€ is different from x.
A similar severity testing can also be performed on the defendant’s statistical evidence that led to a statistically insignificant 1€ damage estimation. Here, the further away we define (positive) x from zero, the higher the probability that the estimated damage of 1€ is different from x. The two opposing results with severity testing at different values of the alternative hypothesis is depicted in the figure below.
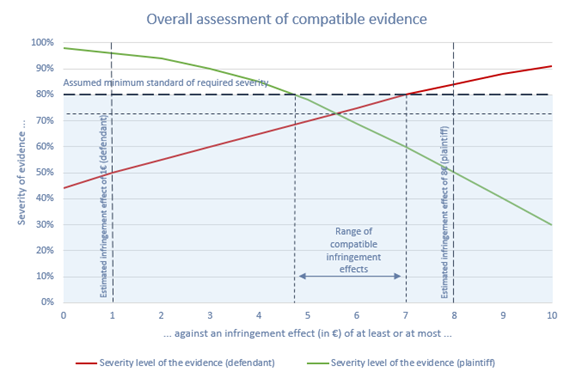
Source: Bönisch-Inderst (2022)
Bönisch and Inderst call this figure the “overall assessment of compatible evidence”. It combines both parties’ estimates on the statistical significance of the cartel infringement effect into one figure based on the severity of their respective sets of evidence. This analysis demonstrates that there may be potential damage estimates x that are compatible with both pieces of statistical evidence at a given minimum standard of required severity level (80% in the example). This minimum threshold is paramount for the compatibility assessment, as it shows the range of values that can or cannot be ruled out by the test. This minimum severity level could be set by the court according to reasonable case-specific circumstances, such as the seriousness of the infringement or the theory of harm.
The compatibility figure above should then be interpreted as follows: if the severity level at the intersection of the two curves is below the assumed minimum standard of required severity, a combined assessment of the statistical evidence of each party might be possible. In this particular case, the set of possible infringement effects lies between approximately 4.8€ to 7€. Instead, if the intersection is observed to be above the minimum standard, it would mean that one party’s estimate weighs with sufficient severity against all the evidence of the other party. Facing this situation, the court should pronounce the provided estimates to be incompatible and the overall evidence at hand to be inconsistent. A closer examination of both parties’ econometric models should then be performed to reveal the reasons behind those contradictions, leading to rejection and re-evaluation of the statistical evidence by the court.
Comment
While the suggestion of Bönisch and Inderst to use severity tests to find a compromise between opposing damages estimations is interesting, it can be applied only if both the claimant’s and the defendant’s estimates are prone to a high level of uncertainty (the authors do mention this in the article3Bönisch, P., & Inderst, R. (2022). Using the Statistical Concept of “Severity” to Assess the Compatibility of Seemingly Contradictory Statistical Evidence (With a Particular Application to Damage Estimation). Journal of Competition Law & Economics, 18(2), p. 414.). In cases where (i) the claimant estimates the relative size of the cartel effect to be large and highly significant or (ii) the defendant estimates a strongly insignificant cartel effect (strongly significant no-effect), it is very unlikely that the methodology will be helpful.
If the severity level at the intersection is above the minimum standard of required severity, the methodology suggests that the estimates of the two parties are not compatible (see above). While this may be the case, it is also highly likely that the true cartel effect lies somewhere on the scale between the claimant’s and the defendant’s estimates. This can be a helpful outcome for judges who, under the EU Damages Directive and its implementation into national law, have the power to estimate damages based on the evidence provided by the parties. Further, even if there is no prospect for a thorough examination of the underlying data and/or appointing a third-party expert to carry out the analysis, the judge should not be discouraged from awarding damages, if the circumstances of the case imply a certain level of likelihood that cartel effects are present.
By Theo Mayer and Akos Reger